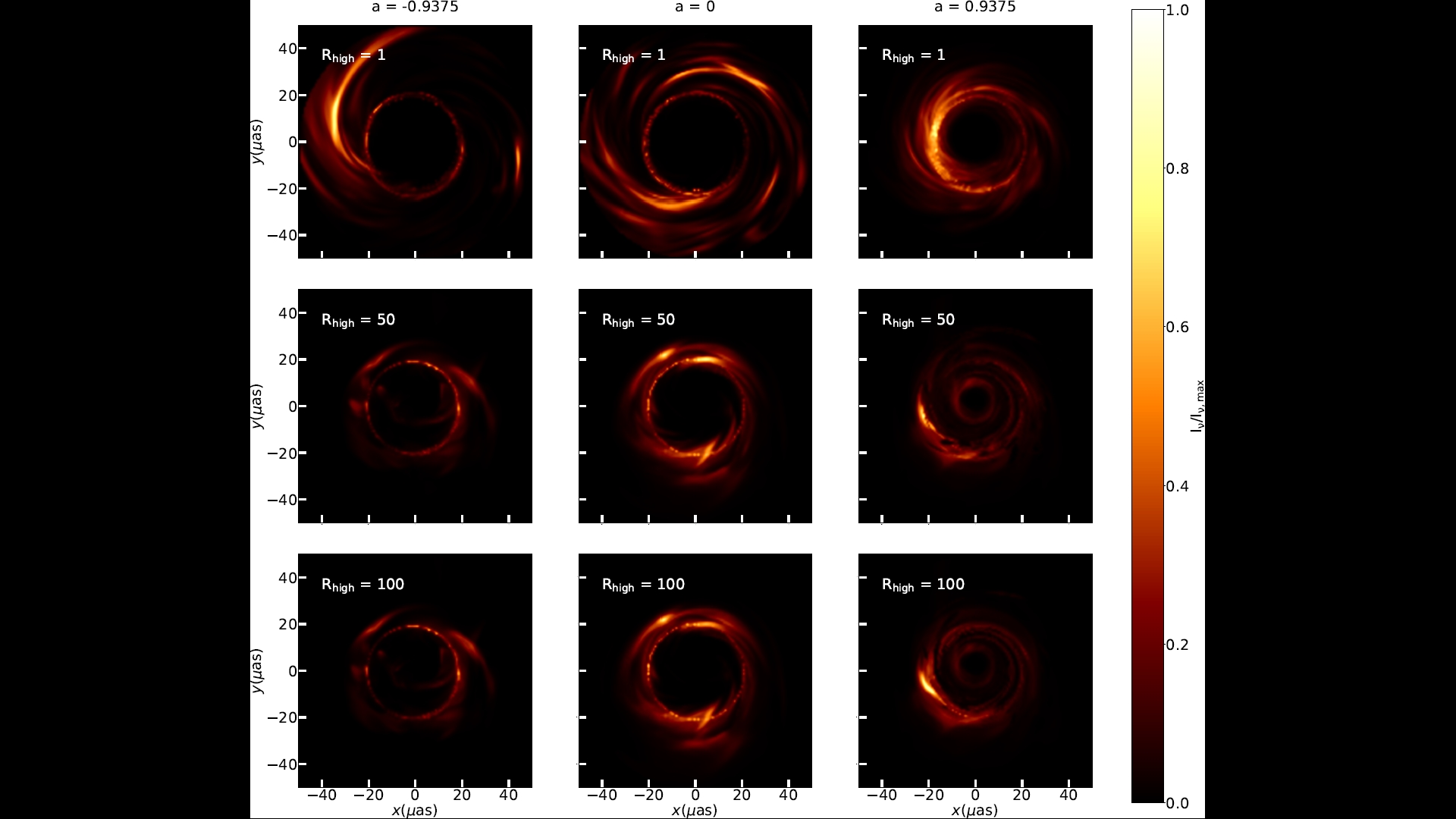
Deep Horizon: A Machine Learning Network That Recovers Accreting Black Hole Parameters
Jeffrey van der Gucht et al. 2020
Deep Horizon is a combination of two machine learning networks that can recover input parameters of images of accreting super massive black holes. In this study, the writers aim to use Deep Horizon to recover physical parameters from images with limited telescope resolution by observing them at higher frequencies. The text describes the characteristics of black holes and the limitations of Earth-based observations and through the use of Deep Horizon, the potential of space-based observations for higher resolutions is explored.
The Event Horizon Telescope (EHT) is a virtual observatory consisting of 8 telescopes spanning the planet. It has released the first image of a black hole shadow in April 2019. EHT images can be used to test the theories of gravity and deepen understanding in plasma physics at event horizon scales. The resolution of EHT images is limited by the size of the earth, which therefore makes it insufficient to determine all parameters of black holes. At the moment, the resolution of EHT is only possible to accurately recover a limited number of parameters, which are the mass and mass accretion rate of SMBHs. Unlike the EHT, the Space-based Very-long-baseline interferometry (SVLBI) allows for higher resolution and improved image quality, which means that it is able to provide more accurate and reliable images. (put time)
The paper acknowledges the data limitations in observational data and emphasizes the use of simulations to generate mock observations of the black hole parameters. Two data sets each comprising 100,000 images were used, which were created with varying frequencies. The images result from post-processing five distinct General Relativistic Magnetohydrodynamics (GRMHD) simulations conducted using the Black Hole Accretion Code (BHAC) and the GRRT code RAPTOR. The GRRT is a training program designed to increase awareness and knowledge of environmentally sustainable disaster response approaches.
The images generated by RAPTOR exhibit a central flux depression at the black hole’s location, surrounded by a bright ring corresponding to the lensed emission ring. This ring scales with the black hole mass and also displays extended emission features from the accretion disk.
Because applying a Gaussian beam on the images can result in the loss of small-scale features, the study investigates the minimum resolution required for reliable parameter estimations by varying Gassian beam widths. In the investigation, it is shown that the network effectively discriminates between spin values up to a 10µas beam width, but at 20µas, accuracy declines. The results show that without a Gaussian beam, the network effectively predicts black hole parameters. With a Gaussian beam, there is a larger scatter on the predictions which indicate that the network is less confident, which also leads to a higher uncertainty. These results show that with an increasing beam width, the uncertainty of the results increase simultaneously.
Through these investigations, the study emphasizes the importance of higher quality training data in machine learning, and that the algorithm’s robustness changes in the amount of training data that is provided. SANE models are mostly used for the accretion flow. SANE models, which stand for “Standard and Normal Evolution”, refers to a type of simulation of how matter falls onto a black hole. Despite the use of SANE models, writers have suggested the potential of using alternative models such as the Magnetically Arrested Disk (MAD) as they acknowledge the current limitations in the training data. To enhance the performance of the investigations, the writers considered a more diverse set of electron temperature models, an increase in the sampling of spin parameters and the use of realistic telescope effects.